Is Human Behavior as Simple as Ant’s?
Posted on November 25, 2021In the world of ants, there is no supervisor of their colony, and worker ants don’t receive orders from their queen. Ants run a decentralized colony with local communication using only 10 to 20 signals, such as alarming, conveying task assignments, and sharing food source locations. Steven Jonson says,
“They think locally and act locally, but their collective action produces global behaviors.”[1]
How about human society? Each individual thinks and acts locally. Like an ant colony, no one person is controlling and authorizing each behavior from above. However, our society is considerably more complex than ant one. From a micro perspective, people act variously with unique intentions and characteristics. No one is the same as anyone else. From a macro perspective, our society is constructed through various individual behaviors such that, even in groups, actions such as working, playing, studying, etc., are still generated by individual agents. Our society is so entangled that it is difficult to understand, leading to wicked problems.
Can we apply the model of ant colonies to help us understand human behavior? Focusing on pedestrians’ motion, like people walking on a street or evacuating from a building, human behavior can be simply described, and we can observe emergent phenomena in the system. In the field of computer crowd simulation, simulated agents walk locally following the prescribed model in the virtual environment as influenced by other agents and objects; this is called a multi-agent system. One of the popular pedestrian behavior models is the Social Force (SF) model, proposed by Helbing.[2] This model describes pedestrian behavior using only physical and psychological forces. Figure 1 shows the acceleration equation of agents: What the equation shows is that each pedestrian’s behavior is further determined by the desired direction and current state and is influenced by other pedestrians, the world, and other objects. Thus, there are only three forces that determine each pedestrian’s behavior.
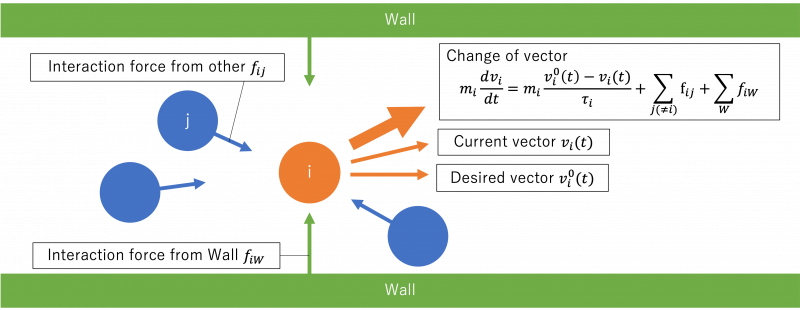
Figure1: Social Force Model[2]
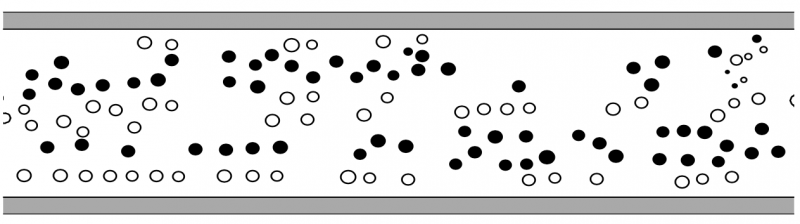
Figure2: Lane formation[2]
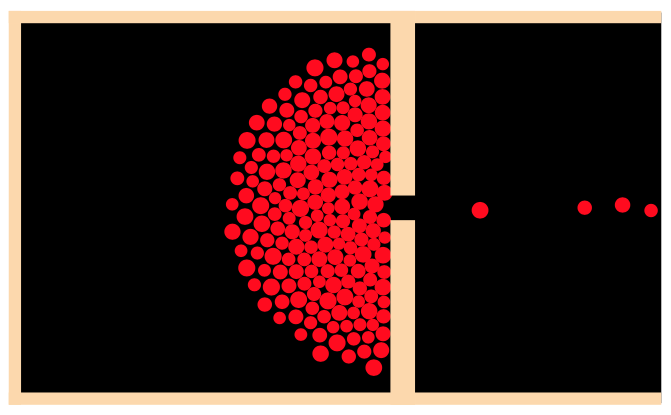
Figure3: Arch-like blocking[3]
By checking such emergent phenomena that can also be observed in the real world, proposed models are validated qualitatively. Suppose we simulate a new situation with an unvalidated model and find problems with the result. In that case, we cannot find out whether the reason comes from the model or simulation setting. For instance, considering an evacuation simulation in a newly designed building, we will find pedestrians stuck in the corridor. Does this happen because the pedestrian model itself has a problem walking in a congested space, or is the width of the corridor too narrow for the building?
On the other hand, by applying this approach to the ant world, we can validate the knowledge about ant roles and behaviors. Suppose something problematic happens in running the ant colony simulation with the ant model developed by current knowledge. In that case, it shows that there is a lack of understanding about the ant world.
By the way, what is sufficient validation? How can we assume the model is well-validated? It is impossible to build a perfect human model that copies the human brain and behavior completely. The SF model is so simple that it cannot perform tricky human behavior such as sudden stops to look at smartphones or attacking others on the street. Therefore, the validation will be conducted in certain situations that suit the purpose of the analysis, and the measure will be that it is convincing for people who evaluate the simulation, such as clients.
There are various usages of crowd simulation. The most popular one is an emergency evacuation such as fire or earthquake. Simulation is used to analyze bottlenecks of a building to escape from fire. In addition, it is also used to analyze a stadium’s leaving scenarios; changing plant arrangement resolves the stock of visitors. In addition, it also evaluates operations such as the order of exit instruction in terms of time and human density.[4]
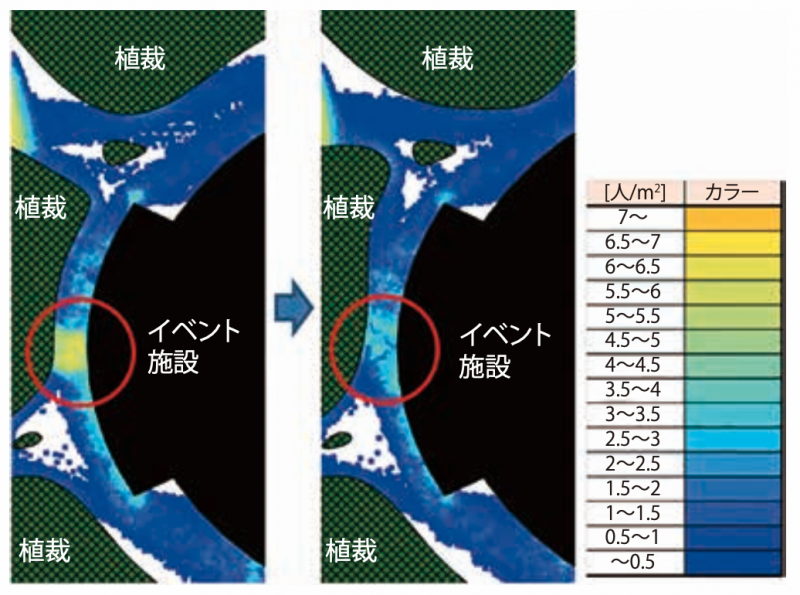
Simulation of the arrangement in studium[4]
Multi-agent simulation is also used in a variety of fields to investigate complex social systems. Schelling’s segregation model, which investigates the mechanism of segregation in the U.S., is also implemented as a multi-agent simulation.[5] Infection of an epidemic is also researched in this field.[6] Actually, some of them were developed before the multi-agent model was proposed. So, it has a chicken-egg relationship affecting each other.
In this way, focusing on the point of view helps researchers to understand complex social systems and human behaviors as interactions among agents like ant colonies. In design studies, changing viewpoints and focusing on specific aspects may help them to explore complex systems and we can
Reference
1. Johnson, Steven. Emergence: The connected lives of ants, brains, cities, and software. Simon and Schuster, 2002.
2. Helbing, Dirk and Peter Molnar. “Social force model for pedestrian dynamics.” Physical review E, 51.5(1995): 4282.
3. Helbing, Dirk and Illés Farkas and Tamas Vicsek. “Simulating dynamical features of escape panic.” Nature, 407.6803(2000): 487-490
4. Inoue, Masako, Hideto Tanaka, and Yoshinobu Adachi. “Progress in Utilizing Crowd Flow Simulation from Architectural Design to Operation : Case Studies from Large Scale Events, Disaster Management, Crime Prevention and Operation.” Journal of Information Processing Society of Japan, 58.7 (2017): 578-581.
5. Schelling, Thomas C. “Models of segregation.” The American Economic Review, 59.2(1969): 488-493.
6. Dong, Wen and Katherine Heller and Alex Pentland. “Modeling infection with multi-agent dynamics.” In International Conference on Social Computing, Behavioral-Cultural Modeling, and Prediction. 172-179. Springer, Berlin, Heidelberg, 2012.